Artificial Intelligence Maintenance
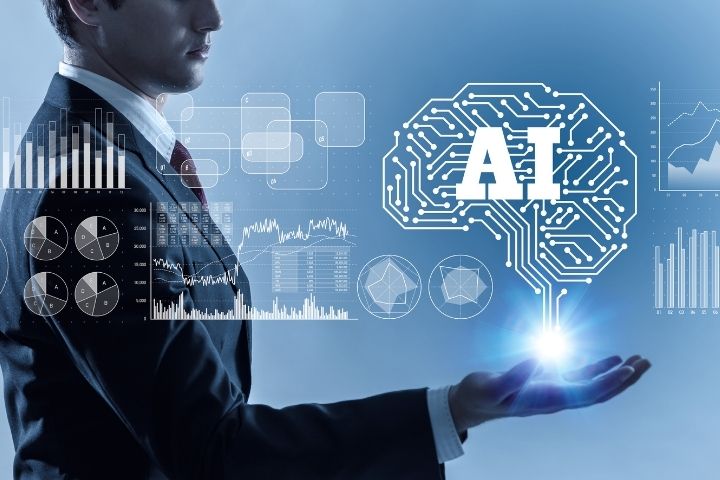
Artificial Intelligence Maintenance, Information obtained from operational data supports an advanced form of predictive maintenance.
Industry 4.0 and Digitization offer the ability to optimize operations and maintenance. Innovations such as digitization, artificial intelligence (AI), machine learning (ML), neural networks, and cloud computing have increased the ability to collect, analyze and monitor equipment health in real-time.
Thanks to this advanced monitoring equipment and analytical methods, engineers responsible for the reliable operation of the equipment can step up the pressure to implement predictive maintenance programs (PdM) to achieve profitability, including:
- optimization of the service life of key equipment;
- minimization of unplanned downtime;
- maintenance cost management;
- Improving the security and operability of the company.
Maintenance can be a profitable center
Energy and chemical/petrochemical production plants are very complex and complicated facilities. Many infrastructure facilities and items manage, contain, and store raw materials and process fluids and gases. Reducing downtime of process units and operations due to unusable equipment and systems is a central point for increasing a company’s profits.
Mechanical failure is the main cause of equipment accidents in the manufacturing industry, while equipment failures lead to 36% of unplanned downtime. Creating more sophisticated maintenance and planning programs increases the level of operational excellence and operability of the equipment.
“Smart” maintenance generates profit
According to a recent McKinsey report, PdM can increase device availability (both process units and devices) by 5 to 15%. Optimized PdM operations extend the life of key equipment by 20 to 40%. More importantly, PdM can effectively reduce maintenance costs from 18 to 25%. Improved monitoring and timely proactive maintenance significantly reduce repair and replacement costs for critical equipment and minimize unplanned downtime and lost productivity. In addition, an unexpected equipment failure can lead to losses greater than the acquisition value of the replaced equipment.
Advantages of PdM
The failure rate of the main equipment in the company
In general, rotary and piston devices have the highest number of failures. Vibration problems are the main causes of failures of rotating equipment, especially pumps. All rotating devices vibrate, but changes in vibration levels over time are indicative of possible problems. In the hydrocarbon processing industry, about 7% of the pumps are used to consume 60% of the money for pump maintenance and repairs. Finding and solving the root causes of vibration or temperature changes thus replaces mere treatment of symptoms. To prevent the recurrence of faults, pump operators must do their utmost to take routine maintenance procedures to the next level. The growing use of intelligent manufacturing strategies and cloud computing will help increase the integrity of PdM activities.
This is not a new concept
Since the 1970s, maintenance workers have begun to install piezoelectric load cells to monitor and detect pump and motor performance problems. Unfortunately, these initial methods encountered continuity problems in data collection. In question, the vibration sensors often operated at different frequencies and amplitudes and had their own initial symptoms.
Only experts could decipher the meaning of the data obtained from the sensors and convert them into usable information. Sensors were often lost or removed during routine maintenance. The primary sensors were physically connected to minicomputers or terminals via wires. Various contaminants, such as dirt and lubricating oils, have degraded the signals of these sensors. The technicians responsible for the reliable operation and maintenance of the equipment found that the initial vibration monitoring methods did not produce the desired results.
Advances in minicomputers, terminals, and portable sensors have contributed to the improvement of device monitoring programs. However, real-time information and data connectivity have remained limited due to computer hardware and software capabilities. Converting vibration sensor signals into usable information continued to be a time-consuming task. In addition, data trends and information were muted in databases and were not easily shared between users.
Remote monitoring equipment methods have been incorporated into preventive maintenance programs. The amount of data collected has never been a problem. In some cases, too much data has reduced the ability to find key information about the health of the facility. It remained a long-term problem to understand what the data obtained indicates the current state of the device. Simply put, you can’t effectively fix something you don’t understand.
Vibration monitoring methods constantly strive to provide and convert collected data into reliable real-time information. All too often, the implementation of regular and preventive maintenance programs reveals a deteriorating condition of the rotating equipment.
Real-time device monitoring
Over the last ten years, the use of wireless technologies, cloud computing, smart devices, and artificial intelligence (AI) has enabled device management through advanced PdM programs. While preventive maintenance is performed based on the manufacturer’s recommended plan, PdM takes maintenance activities to the next level. In PdM, real-time process and technology data generate trends and histories used to predict process equipment changes. Extending equipment uptime and shortening production processes through improved reliability/maintenance programs, such as advanced PdM technology, help increase the level of operational excellence and safety of the entire enterprise.
Advanced PdM analysis
For PdM programs to be effective, they require the presence of thorough and valid data, including analytical tools; this is the only way to decide on the basis of available information. Recent advances in AI and ML (machine learning) make it possible to analyze and convert large amounts of collected data into patterns. As part of rotary and piston device monitoring, advanced vibration sensors use cloud computing to record real-time data in a variety of formats. Innovative AI and ML algorithms, built on a combination of software and neural networks, convert and analyze wireless sensor data.
This information generates trends that identify normal and unhealthy operating activities. More importantly, AI algorithms “learn” from transmitted measured vibration data and distinguish between “normal” or unacceptable signals.
A performance relationship between key devices is possible using predictive analysis based on AI, ML, and neural networks. These validated analytical tools are used to identify the real causes of performance variations in rotary and piston equipment. Early fault identification allows optimal corrective action to be taken before significant equipment damage or failure occurs, minimizing repair costs, reducing unplanned downtime, and ensuring safe operation.
Visual or routine condition checks cannot observe deteriorating conditions of pumps and compressors. The AI and ML algorithms identify patterns from history and detect performance degradation, as evidenced by variations in device trends. Equipment performance problems are identified much earlier than through traditional preventive maintenance methods. System-generated alarms alert maintenance technicians that further investigations are required.
AI-based predictive analysis also goes beyond mere failure reporting. Data and operating history are used for the failing equipment or component’s residual life estimate (RUL). Thanks to RUL, maintenance and reliability technicians have complete information available for repair planning and equipment replacement, which in turn has a minimal impact on the uptime of the entire process.
Data visualization
Unspecified data has only a limited value. The basis of PdM is data visualization. Advanced PdM uses AI-based analytical and neural networks to convert collected data into usable information. They usually include dashboards that allow users to see device status data and track trends quickly. With such graphics, technicians are able to easily interpret the health of the equipment/process unit and make more informed data-driven decisions. In addition, RUL estimates are summarized in a graphical form to provide centralized information.
Secure wireless technologies and mobile application connect advanced sensors to the cloud for analysis using AI and ML software. With full industrial Internet of Things (IIoT) and cloud computing applications, maintenance technicians can constantly monitor the health of process-critical devices. The ability to predict RUL and “time to failure” is invaluable. With such information, service and operations groups can schedule repairs in advance instead of just responding to an emergency shutdown or unplanned outage.
Case study: L&T Nabha power plant
L&T Nabha Power Plant is the first supercritical coal-fired power plant and one of the most efficient power plants in India. This facility operates two 700MW supercritical heat units and is a major electricity provider in the state of Punjab in northern India. As a major energy provider, the reliability of the Nabha power plant is a key issue for the region. The unplanned need for maintenance and shutdown of this power plant unit has a dramatic and adverse effect on the productivity and profitability of regional companies and residential customers. Unfortunately, there were three unplanned downtimes in this power plant in one year due to pump accidents.
This condensate pump experienced lingering problems with cavitation erosion and excessive vibration, which led to bearing failures and unplanned failure of the Nabha power plant unit.
In the field of electricity generation, pumps are a key technological device. The condensate pump is one of the pumps that play a crucial role in maintaining the steady operation of the equipment (Fig. 4). It is a horizontal vane pump with an output of up to 1,650 m³ / h with a discharge of 9 MPa (62 psi) at 986 rpm. Every day this pump is down, the plant loses up to $ 250,000 in lost revenue. As a result of unplanned maintenance or failure of this pump, the cost of repairs exceeds tens of thousands of dollars.
Condensate pumps very often experience unplanned downtime due to failures due to cavitation erosion. As the primary electricity provider in the region, the reliability of the condensate pumps was a top priority.
To prevent fault conditions and increase the operability of the entire unit, the plant’s responsible engineers decided to install real-time vibration monitoring and an advanced AI-based predictive analysis solution for all condensate pumps. The new monitoring strategy focused on the early detection of a pump and component failure. In addition to fault detection, this monitoring solution included AI-based algorithms that provide reliable RUL estimates before subsequent outages. Several advanced wireless monitoring sensors have been installed to address bearing failures with these condensate pumps:
- bearings on the driven side of the electric motor;
- bearings on the drive side of the electric motor;
- bearings on the drive side of the pump;
- bearings on the driven side at the pump.
Figure 5: Taking into account previous work orders for maintenance service work, the responsible technicians have chosen the optimal location for the state-of-the-art acoustic vibration sensors
The monitoring system used secure Wi-Fi sensors to collect and record measured vibration data via the cloud continuously. Cloud-based AI algorithms analyzed and processed the collected data.
Approximately six weeks after installing the advanced sensors and analytical system, the new monitoring program alerted maintenance personnel that the blade had failed. This resulted in cavitation erosion at the condensate pump. Maintenance personnel verified this fault with a portable vibration monitoring device and performed a partial disassembly to confirm damage to the pump blades visually. Damaged blades were temporarily repaired before the pump was put back into operation.
Thanks to the application of AI-based predictive capabilities as well as advanced vibration monitoring functions, the L&T Nabha power plant unit has avoided serious pump failure and unplanned downtime. Using an advanced AI-based PdM system, the remaining life before full failure was estimated at 25 days. This created sufficient time to schedule a pump replacement during an already scheduled maintenance intervention. The early intervention reduced the number of repairs needed and minimized interruptions in the operation of the entire unit.
It’s not just black boxes.
IIoT, cloud computing, and wireless technologies support AI-based data analysis as part of an advanced PdM program. With full use of AI and ML methods, engineers can detect anomalies or faults in key equipment well in advance of the failure mode. Advanced wireless vibration / acoustic sensors support PdM programs for real-time data collection and recording.
Figure 6: Advanced wireless acoustic sensors have been installed with a strong magnet and two-component epoxy to withstand the demanding operation of this pump
Using AI, ML, and neural network algorithms, advanced analytical tools develop historical trends of monitored devices or components. With a complete operational history, AI-based analytical tools identify changes in trend data and estimate the RUL of the monitored equipment. With RUL, maintenance personnel are able to take corrective action well before the failure itself and thus focus on keeping the equipment in safe operating mode. Advanced PdM programs support improved results-based maintenance schedules that extend equipment uptime and increase operational safety.
Also Read : Unlock The Potential Of Hybrid Artificial Intelligence